Data Validation for Machine Learning
(This article originally appeared on KDNuggets.com here. For more, visit https://www.kdnuggets.com/)
While the validation process cannot directly find what is wrong, the process can show us sometimes that there is a problem with the stability of the model.
Data is the sustenance that keeps machine learning going. No matter how powerful a machine learning and/or deep learning model is, it can never do what we want it to do with bad data. Random noise (i.e. data points that make it difficult to see a pattern), low frequency of a certain categorical variable, low frequency of the target category (if target variable is categorical) and incorrect numeric values etc. are just some of the ways data can mess up a model. While the validation process cannot directly find what is wrong, the process can show us sometimes that there is a problem with the stability of the model.
Train/Validation/Test Split

The most basic method of validating your data (i.e. tuning your hyperparameters before testing the model) is when someone will perform a train/validate/test split on the data. A typical ratio for this might be 80/10/10 to make sure you still have enough training data. After training the model with the training set, the user will move onto validating the results and tuning the hyperparameters with the validation set till the user reaches a satisfactory performance metric. Once this stage is completed, the user would move on to testing the model with the test set to predict and evaluate the performance.
Cross Validation
Cross Validation is a technique to assess the performance of a statistical prediction model on an independent data set. The goal is to make sure the model and the data work well together. Cross validation is conducted during the training phase where the user will assess whether the model is prone to underfitting or overfitting to the data. The data to be used for cross validation have to be from the same distribution for the target variable or else we can mislead ourselves as to how the model will perform in real life.
There are different types of Cross Validation, such as:
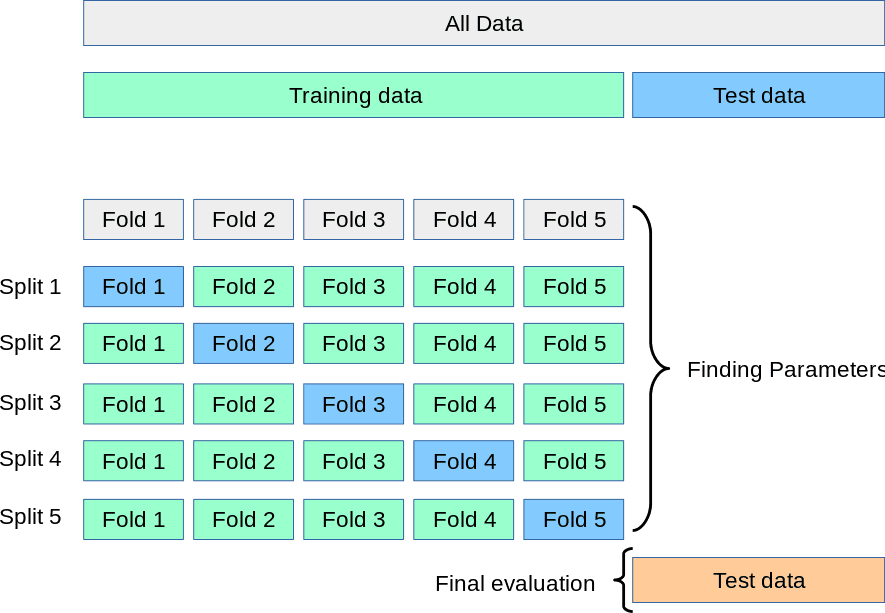
.

- K-fold Cross Validation
- In the circumstance that we would like to preserve as much data as possible for the training stage and not risk losing valuable data to the validation set, k-fold cross validation can help. This technique will not require the training data to give up s portion for a validation set. In this instance, the dataset is broken into k number of folds wherein one fold will be used as the test set and the rest will be used as the training dataset and this will be repeated n number of times as specified b the user. In a regression the average of the results (e.g. RMSE, R-Squared, etc.) will be used as the final result. In a classification setting, the average of the results (i.e. Accuracy, True Positive Rate, F1, etc.) will be taken as the final result.
- Leave-One-Out Validation (LOOCV)
and the dataset will be split into n-1 data sets and the one that was removed will be the test data. performance is measured the same way as k-fold cross validation.
- Leave-One-Out Validation is similar to the k-fold cross valiadtion. The iteration is carried out n specified times
Validating a dataset gives reassurance to the user about the stability of their model. With machine learning penetrating facets of society and being used in our daily lives, it becomes more imperative that the models are representative of our society. Overfitting and underfitting are the two most common pitfalls that a Data Scientist can face during a model building process. Validation is the gateway to your model being optimized for performance and being stable for a period of time before needing to be retrained.
Stay in the loop.
Subscribe to our newsletter for a weekly update on the latest podcast, news, events, and jobs postings.